Earth system science aims to establish the scientific basis for the development of a sustainable stewardship of the Earth system under global change.
With the advent of satellite-based remote sensing, in-situ global observation systems, wireless sensor networks, and citizen science projects, the geoscience community has now access to an unprecedented amount of data to develop such a science and operational basis in combination with a novel generation of Earth system models.
How to deal with the wide heterogeneity of data types, data quality, and data accessibility poses both methodological and technical challenges. Since the observations are mostly only indirectly linked to the variables and parameters of interest, their interpretation relies on their integration or assimilation with model-based methods. Currently, a lot of effort is put into integrating biogeochemical with geophysical and geofluiddynamical models in order to account for the various non-linear feedback mechanisms between geo-physical, biological, and chemical processes on the systems scale. This requires to consider states and fluxes on a wide range of spatiotemporal scales, and hence implies the necessity for tailored upscaling techniques, which in return pose a major model reduction challenge. The promising combination of novel high-resolution data-analysis methods with machine learning techniques is still a largely unexplored avenue. The use of data assimilation methods in mechanistic and inverse models is another example of the synergisms that emerge from combining data science and computational science.
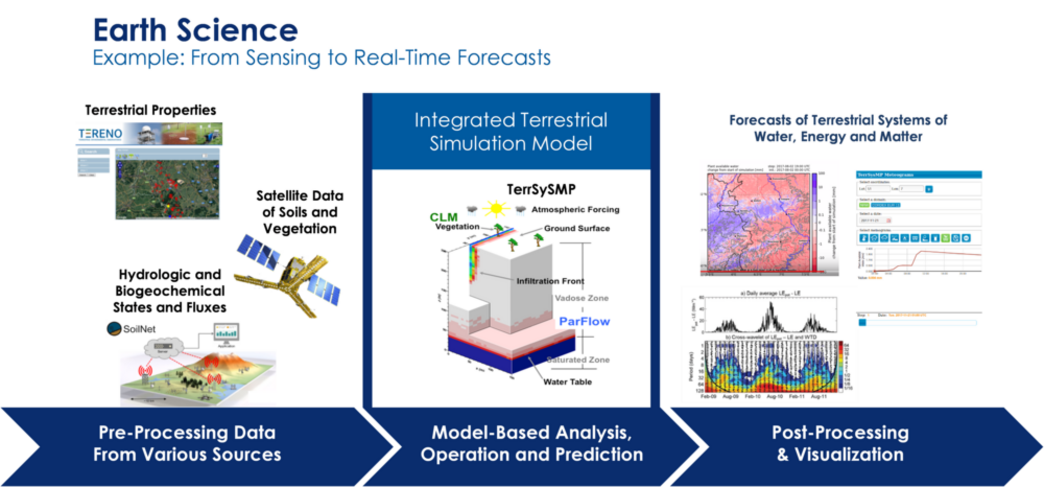