Sophia Münker successfully defended her dissertation
Congratulations to Sophia Münker for the successful defense of her dissertation on "Generic Importance Sampling via Stochastic Optimal Control and Dimensionality Reduction for Stochastic Reaction Networks".
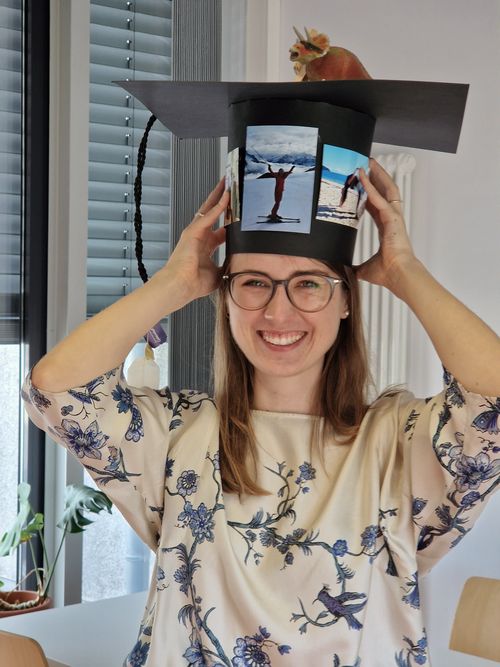
Sophia Münker successfully defended her thesis on "Generic Importance Sampling via Stochastic Optimal Control and Dimensionality Reduction for Stochastic Reaction Networks".
The abstract of her thesis is as follows:
"A Stochastic Reaction Network (SRN) is a stochastic process applied to model many real-life phenomena, including the time evolution of biological and chemical systems in which a small copy numbers of molecules interact with each other through reactions. In this thesis, we aim to improve the efficiency of Monte Carlo (MC) estimators for deriving statistical quantities in SRNs, particularly focusing on rare event probabilities. Therefore, we propose a parameterized Importance Sampling (IS) scheme. The optimal IS parameters minimize the resulting MC estimator's statistical error and are determined by solving a dynamic programming equation or a Hamilton-Jacobi-Bellman (HJB) equation. Since determining the optimal IS parameters for higher-dimensional SRNs (i.e., SRNs with multiple interacting species or molecule types) suffers the curse of dimensionality, we develop two approaches to tackle this issue. The first approach reduces the number of parameters by introducing an ansatz function for which the parameters can be determined by gradient-based stochastic learning (e.g., gradient descent). For the second approach, we develop the Markovian Projection (MP) technique, which can be applied to reduce the dimension of an SRN while preserving the marginal distribution. We derive a near-optimal IS estimator by solving the HJB equation for a lower-dimensional MP-SRN and mapping the resulting IS parameters back to the original full-dimensional SRN. Beside the application of MP for IS, we explore the opportunities of the dimension reduction approach for deriving the quantity of interest via a control variate estimator and via solving the Kolmogorov backward equation for a lower-dimensional MP-SRN."