Alper Yegenoglu successfully defended his dissertation
Congratulations to Alper Yegenoglu for the successful defense of his dissertation on "Gradient-Free Optimization of Artificial and Biological Networks using Learning to Learn"
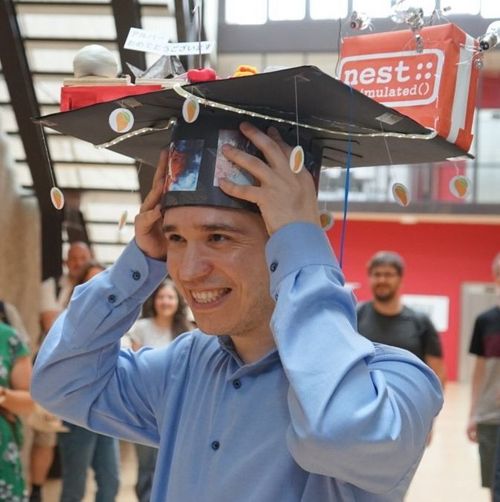
Alper worked in the intersection of machine learning and computational neuroscience. Alper focused his research on biological and artificial neural network learning. In artificial intelligence, gradient descent and backpropagation are commonly used to optimize neural networks. However, applying these methods to biological spiking networks (SNNs) is challenging due to the binary communication scheme via spikes.
Alper investigated gradient-free optimization techniques employed on artificial and biological neural networks. He utilized metaheuristics like genetic algorithms and the ensemble Kalman Filter (EnKF) to optimize network parameters and trained them for specific tasks. This optimization scheme was integrated into the concept of learning to learn (L2L), which involves a two-loop optimization procedure.
Alper applied initially the gradient-free methods on artificial networks and extended it to biological networks, specifically to spiking reservoir networks, and multi-agent systems. By analyzing network parameters and their hyper-parameters, he gained insights into the optimization process.
Through his work, he demonstrated the effectiveness of gradient-free optimization techniques by utilizing learning to learn.