Daniel Wolff successfully defended his dissertation
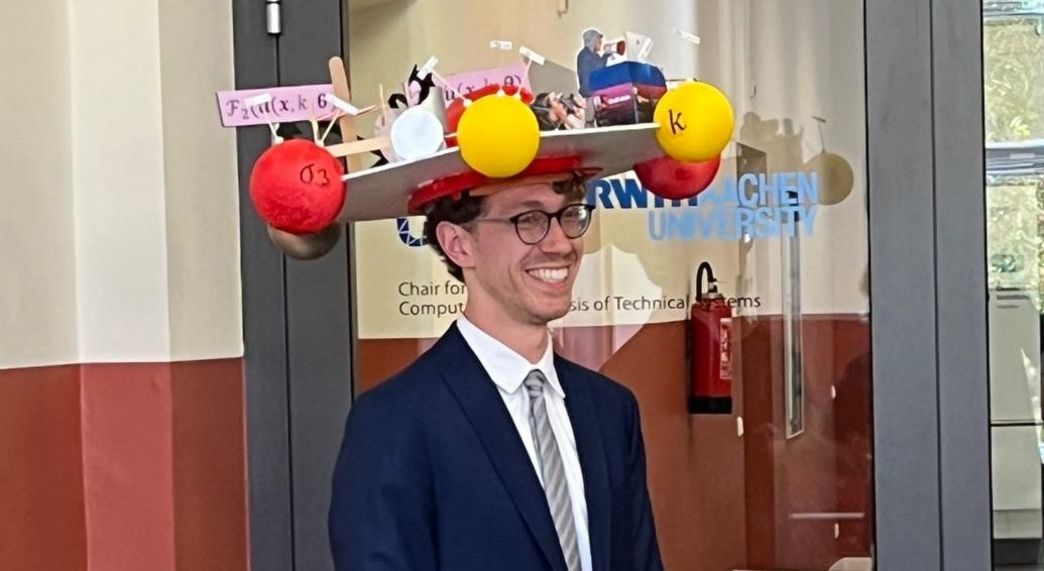
Congratulations to Daniel Wolff for the successful defense of his dissertation on "Learning-Based Approaches for the Analysis and Optimization of Profile Extrusion Dies and Bioreactors".
Daniel Wollf successfully defended his thesis "Learning-Based Approaches for the Analysis and Optimization of Profile Extrusion Dies and Bioreactors". The reviewers were Prof. Dr. Stefanie Elgeti (TU Wien and RWTH Aachen), Prof. Marek Behr, Ph.D. (CATS at RWTH Aachen) and Prof. Elie Hachem, Ph.D. (MINES University ParisTech) while the committee was chaired by Prof. Dr. Julia Kowalski (MBD at RWTH Aachen).
In his thesis, he investigated how methods from the field of machine learning can be incorporated into classical engineering workflows and thus provides another contribution toward bridging the gap between these two disciplines. Precisely, he examined how Machine Learning algorithms can enhance simulations, i.e., by reducing computing times, to enable challenging tasks like the optimization of even complex industrial applications. While being of interest in many engineering disciplines, e.g., the design of profile extrusion dies or bioreactors, repeatedly evaluating expensive high-fidelity (or full-order) simulation models is usually infeasible despite the advances in computational powers during the last decades. Machine learning algorithms are promising candidates to overcome this drawback as they --- once trained during an offline phase --- allow for rapid evaluations online, e.g., within an optimization loop.
In the first part of his thesis, he considered physics-informed neural networks to create reduced-order models for simulating the flows in bioreactors and profile extrusion dies. By incorporating knowledge about the problems' governing equations into the construction process of the reduced simulation model, superior accuracy and interpretability compared to classical black-box machine learning approaches are expected while simultaneously obliterating the need for data. He presented two novel general strategies that enable physics-informed neural networks as reliable reduced models and demonstrate them on the considered application cases.
In the second part of his thesis, he focused on parametric shape optimization and study reinforcement learning: The use of reinforcement learning agents which make decisions based on (partial) observations of a system's state as an alternative to classical optimization algorithms allows for two approaches, i.e., incremental and direct shape optimization. On two different profile extrusion die geometries with differing design objectives, he compared both approaches and discuss the performance of different learning algorithms based on their classification. Finally, he explored vectorized environment training as one possibility of how learning the decision rule can be accelerated by parallelization.